A guest post by Dr. Lars Kai Mäder | 11/22/24
Measuring success in marketing is more complex than ever. More touchpoints, more channels and less and less measurable information about the exact customer journey. Use a practical example to find out how an efficiency analysis is possible using a marketing mix model and how the advertising budget can be optimally determined.
The crux of measuring success in marketing
Advertisers have always wanted to know how successful their advertising measures are. Measuring KPIs is the basis for optimization and sensible budget use. John Wanamakera 19th century economist and businessman and father of modern advertising, coined the phrase:
Half the money I spend on advertising is wasted. The problem is, I don’t know which half.
With the Internet and developments in online marketing, Wanamaker’s problem initially seemed to be at least partially solved. Clicks and conversions were easily measurable and the success of each advertisement could be directly attributed. Unfortunately, that is a thing of the past. With the emergence of a multitude of online channels, complex customer journeys, blocking of third-party cookies, GDPR, ad blockers, device changes and much more, measuring success is now much more difficult. Nevertheless, it is essential for allocating budgets sensibly, achieving desired goals and asserting oneself in a challenging environment. Today, no advertiser can afford gut feeling or chance.
What a marketing mix model can achieve
Marketing Mix Modeling (MMM) is a statistical analysis model. It is based on aggregated advertising expenditure as well as sales and sales figures and is intended to determine the optimal allocation of future advertising expenditure. Based on a training data set, a model is created that determines the optimal distribution of the future marketing budget across the individual channels. The effects of various marketing activities and external influences on the target key figure are incorporated into the model.
A big advantage of the model is that no personal data is required. This avoids all discussions about third-party and first-party cookies. All necessary data, such as past advertising expenditure and sales figures generated, are completely available within the company.
In particular, the interactions between the individual channels as well as hidden effects can be determined using MMM. For example, channels to which only a few direct conversions were attributed can prove to be very influential.
Practical example of marketing mix modeling
Marketing mix modeling is an iterative process. After data analysis and optimization, the model is first checked and adapted to the new data situation. The model cycle is continuously corrected and adapted to the circumstances. The frequency of review and adjustment depends on the customer, their requirements and goals.
Using a real example, we will examine the individual steps in detail. The company, a manufacturer in the fashion sector, sells its products both offline and online, on various digital marketplaces and in its own online shop. In this particular case, however, only online sales and advertising expenditure were analyzed; accompanying offline measures were not taken into account.
Objective
Marketing mix modeling is about understanding the effectiveness of advertising, its efficiency and, based on this, the optimal budget allocation. However, the advertiser must determine which target metric this interaction should be analyzed against. As a rule, including in our practical example, this target key figure is sales.
Recording the initial situation
A thorough recording of the initial situation and collection of all relevant data is the basis for meaningful modeling. The data collection period should be as long as possible, ideally more than two years. In our example, however, the survey period was only 22 months.
In our case, the recorded data, sales and all marketing costs, are broken down in detail by channel. With regard to marketing costs, the data was available separately according to Google Ads, Microsoft Ads, Paid Social and a special budget called Brand Digital. The main share was on Google Ads, which is why this output block was split into brand, generic and shopping. In addition to the specific expenditure on these advertising channels, figures for the immediate advertising reach, ie the number of clicks, were also available. In addition, marketing measures without costs such as newsletters and their recipient numbers were recorded. Ideally, all marketing expenses are available to the model, including offline marketing measures such as print, radio/TV and ooH. However, this was not possible in this specific case and the analysis only took online marketing measures into account.
Accompanying information from the company, which was also collected in the practical case, was promotional campaigns and the corresponding pricing policy at the respective time.
In addition to the marketing factors, we also look at contextual factors that are intended to reflect the advertising environment. For enrichment purposes, in our case results of the Google trend analysis for the core category were recorded as well as economic development, sales periods and visibility in organic search.
Ultimately, it is important to collect as much data as possible so that the model has a good basis and the other influencing factors that are not taken into account have a negligible impact.
Modeling
The mountain of information collected from various sources must now be made comparable and analyzable. To do this, the data formats must be aligned and the data converted into a common time series format. In the practical case, the data is aggregated at the weekly level.
In a first step, the algorithm estimates the influence of the individual factors stored in the model. The statistical key figure R2 is primarily used to assess the quality of the model, with all values above 0.8 being considered good. In our specific case, the key figure was 0.85. The model fit can also be illustrated using a graphic. Figure 1 compares the actual weekly sales figures (orange) with the sales figures estimated by the model (dashed blue). Since the blue line estimated by the model follows the line of the actual sales figures over long stretches of time, one can assume that the model works.
Analysephase
In addition, in the first step, the specific effects of the individual advertising channels are determined and their effectiveness is estimated in terms of the advertising budget used. In our case, Paid Social and Google Ads Shopping, among others, showed large discrepancies between their share of spending and the advertising effects attributed to these channels (Figure 2). Paid social has significantly more benefits than it costs. With Google Ads Shopping the opposite is the case, the costs significantly exceed its benefits.
Recommendation
In a second analysis step, concrete optimizations for the allocation of the advertising budget can now be derived from the model created. How should the budget distribution be changed to maximize the actual target, sales? These optimization models can be run for different budget levels. The budget variance of the individual channels can also be limited in order to increase the acceptance of the changes in the decision-making committee.
Let’s take our practical example in Figure 3. The gray bars represent the costs and sales that actually occurred during the analysis period. The light orange block shows how sales could develop if, with the same costs, the expenses in the channels could deviate up or down by 20 percent. Finally, the third block shows the sales with an allowed deviation of 60 percent. With an unchanged budget, significant sales increases of 17 percent can only be achieved by changing the budget allocation within the marketing channels.
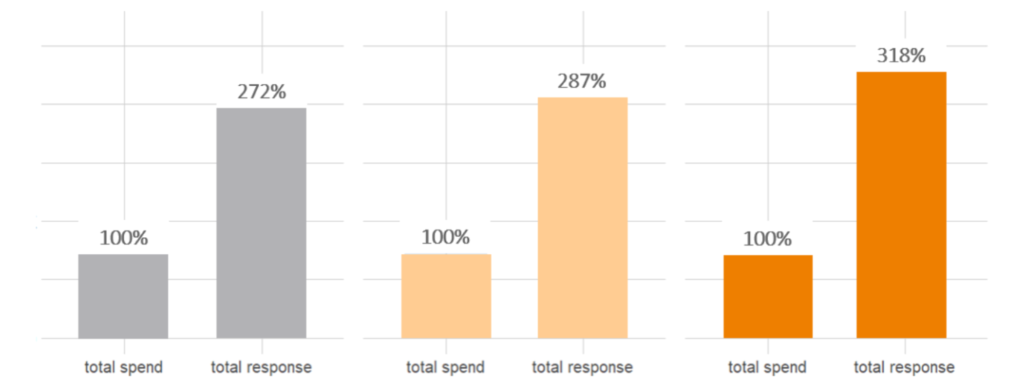
The level of recommended change is listed individually for each channel. For example, in the practical case, the paid social channel accounted for 4.4 percent of the costs and accounted for 10.5 percent of sales. With an allowed variance of up to 60 percent, expenses rise to 7 percent of the marketing budget and are responsible for 10.3 percent of much higher sales.
Who can benefit from marketing mix modeling?
In this practical example, the results are very impressive. The model has proven itself and shows enormous potential for improvement in the future by using the channels differently. In order for success to be determined so clearly through marketing mix models, a few prerequisites are necessary. The quantity of data must be very high, there must be a lot of transactions and several marketing channels must be in use. The period under consideration should also be as long as possible, ideally more than two years.
The less and the worse the data, the less accurate the model will be. A marketing mix model will not be suitable for companies with low volumes and a focus on a few customers. Even small online shops have to consider whether the data is sufficient and there is enough scope for optimization. Manufacturers and shops that record high transaction numbers, possibly operate multiple sales channels and use large marketing budgets in multiple channels can achieve meaningful results.
More efficiency in the marketing mix with meaningful analysis
The statements of a marketing mix model are verifiable, concrete and measurable. Given the complexity of today’s customer journey, the diversity of marketing channels and the decreasing availability of personalized data, a perfect planning and analysis tool is urgently needed. This is the only way to increase the overall efficiency of advertising expenditure and to make unused potential visible. The prerequisite for a meaningful model is extensive and careful data collection and analysis. Once this homework has been done, marketing mix models provide concrete assistance for target-oriented marketing and are a source of competitive advantage. They provide at least an approximate answer to John Wanaker’s question about which half of the marketing budget has been wasted so far or ensure that this half is also used profitably.
Google introduces open source marketing mix model Meridian
Source: onlinemarketing.de