A new chip dedicated to artificial intelligence is gradually being introduced into your laptop. What is it for? Do you really need it? Our explanations.
Two letters have been on everyone’s lips since 2022: AI. Artificial intelligence is breaking out of the confines of science fiction to become an integral part of our lives in real-world applications. Our smartphones, computers, tablets and even game consoles are now equipped to handle computational tasks related to AI that are more or less demanding.
Chips have been introduced into our laptops, the NPUs (Neural Processing Unit), computing units that are much more efficient than your processor at processing AI requests. We tell you in concrete terms what they are used for and why they are now at the heart of consumer computing.
A new chip in our computers
Before getting into the heart of the matter, it is necessary to define what an SoC is, of which the NPU, this new chip, will be an integral part. SoC, for System-on-Chip, is a term associated with smartphones, tablets and other portable PCs.
Instead of putting several discrete chips on a printed circuit board as in the past, everything is integrated into a single circuit thanks to miniaturization: the processor (CPU), the graphics chip (GPU), the modem, the audio. Sometimes, an SoC is also dedicated to other tasks like Apple’s T2 for security in Mac computers.
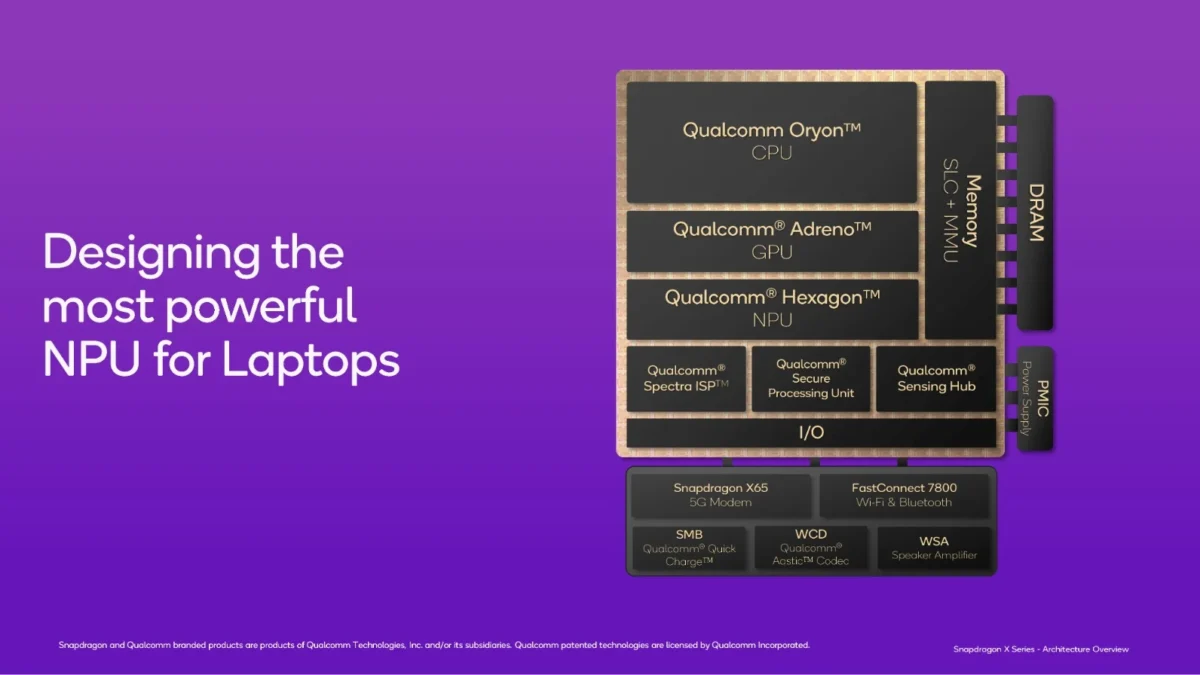
But these SoCs are likely to evolve according to the uses and technologies that manufacturers want to integrate into them. AI is arriving on our smartphones, but also our laptops and it is becoming necessary to perform some of these treatments directly on the device. We are talking here about running natural language models like GPT through Copilot on Windows, performing processing or even image generation or even automatic subtitles and various accessibility features.
What is the point of an NPU?
As these AI and machine learning were able to partly operate locally, on devicethanks to the integration of dedicated chips, NPUs, for Neural Processing Units. At Apple we call it the Neural Engine, and at Google we call it the Tensor Processing Units for TPUs, but the goal is the same: to accelerate AI-related workloads.
Your processor and graphics card are capable of performing machine learningbut have their strength and weakness in this regard. An NPU is dedicated to simulating a massive amount of data in parallel, to mimic the operation of neural networks, which perform the same operation (matrix or vector) millions of times, but with different parameters.
The CPU can focus on a single inference task, but at a very low latency, while the GPU, thanks to AI accelerator chips (notably the Tensor Cores of Nvidia RTX graphics cards), can also process a massive amount of data in parallel, but for a much higher energy consumption. The NPU still lags far behind GPUs for big inference jobs, such as running a real LLM locally or performing advanced image generation, and this is because of the huge difference in terms of TOPS and computational precision.
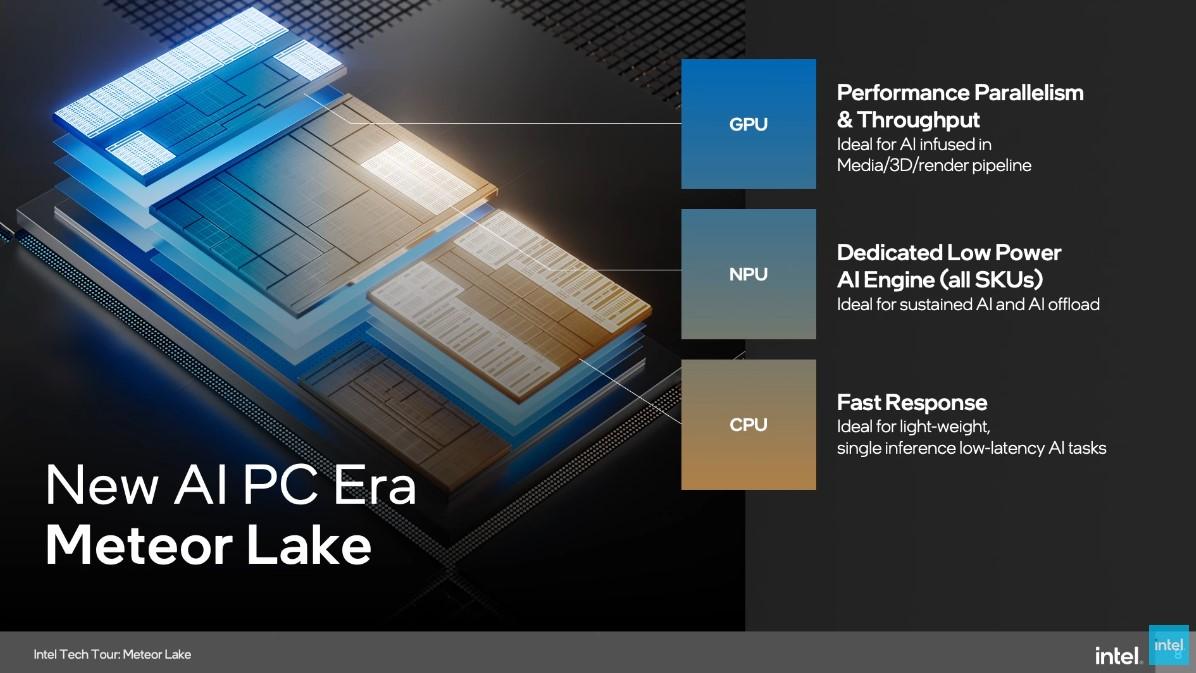
The raw power of an NPU is calculated in TOPS, for Trillions of Operations per Second, similar to TFLOPS for GPUs. Thus, the NPUs of current SoCs oscillate between 40 and 50 TOPS, some consumer graphics cards far exceed 1000 TOPS, but for a much higher consumption.
It is also necessary to be aware of the computational precision of these chips. Indicated in the form of integers and bits, this value represents a compromise between speed and precision. Thus, INT4, the smallest precision, can be useful for tasks where speed of execution is a priority.
The INT8 value is generally favored by manufacturers to calculate the raw power of an NPU, offering superior calculation precision for a calculation speed high enough for our uses, such as image recognition as well as basic photo/video processing.
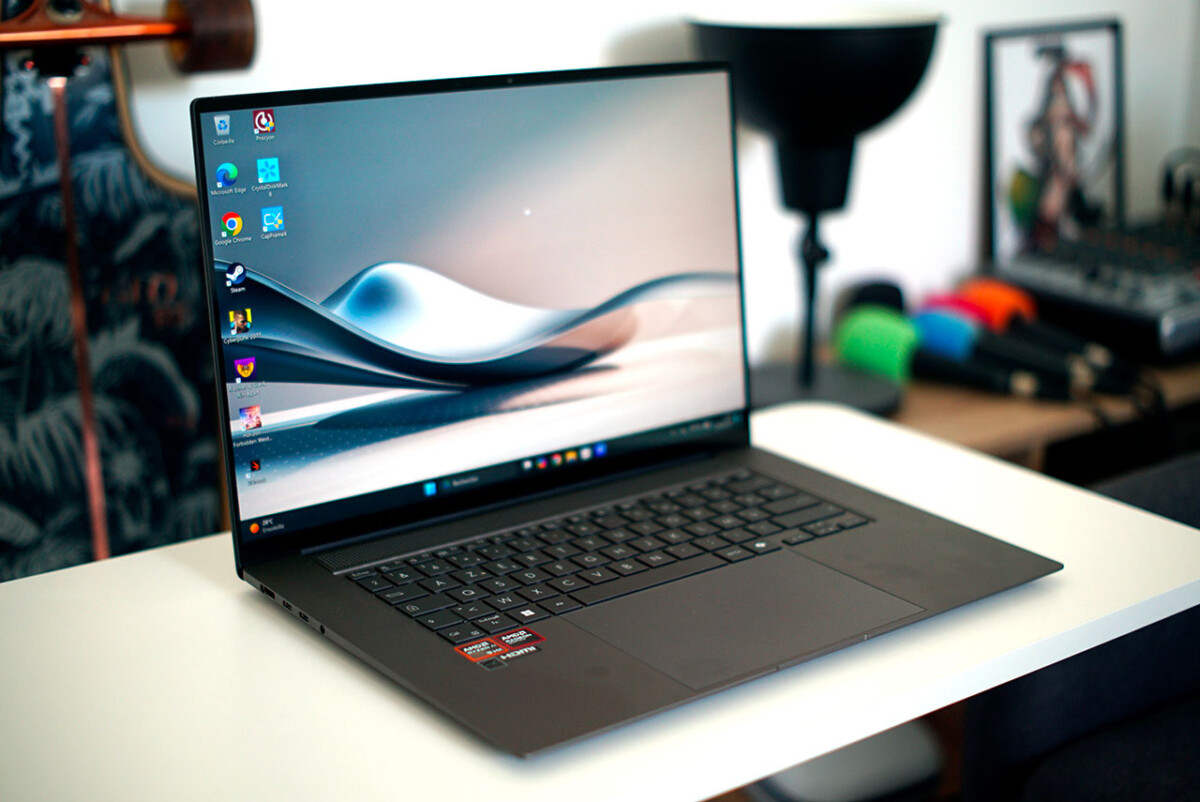
Generally, manufacturers reason in INT8, because it is a sufficient precision for general public uses, but professionals and scientists will prefer precisions in 16 and even 32 bits according to their needs. These values are more precise, but otherwise more demanding in raw power.
What use in our machines?
For users, it’s about latency and security. Some AI tasks that are offloaded to the NPU are processed faster locally than in the cloud, depending on the robustness of the chip. So, this or that task will work better locally on the NPU or in the cloud depending on the chosen workload.
An NPU will really offload the work of the CPU and GPU for lighter tasks, like live translation, automatic Windows subtitles or the camera effects that we now see on Microsoft Teams or Google Meets. Microsoft assures that the NPU is 100x more efficient on Windows on all these tasks than the CPU, with therefore gains in autonomy to the key.
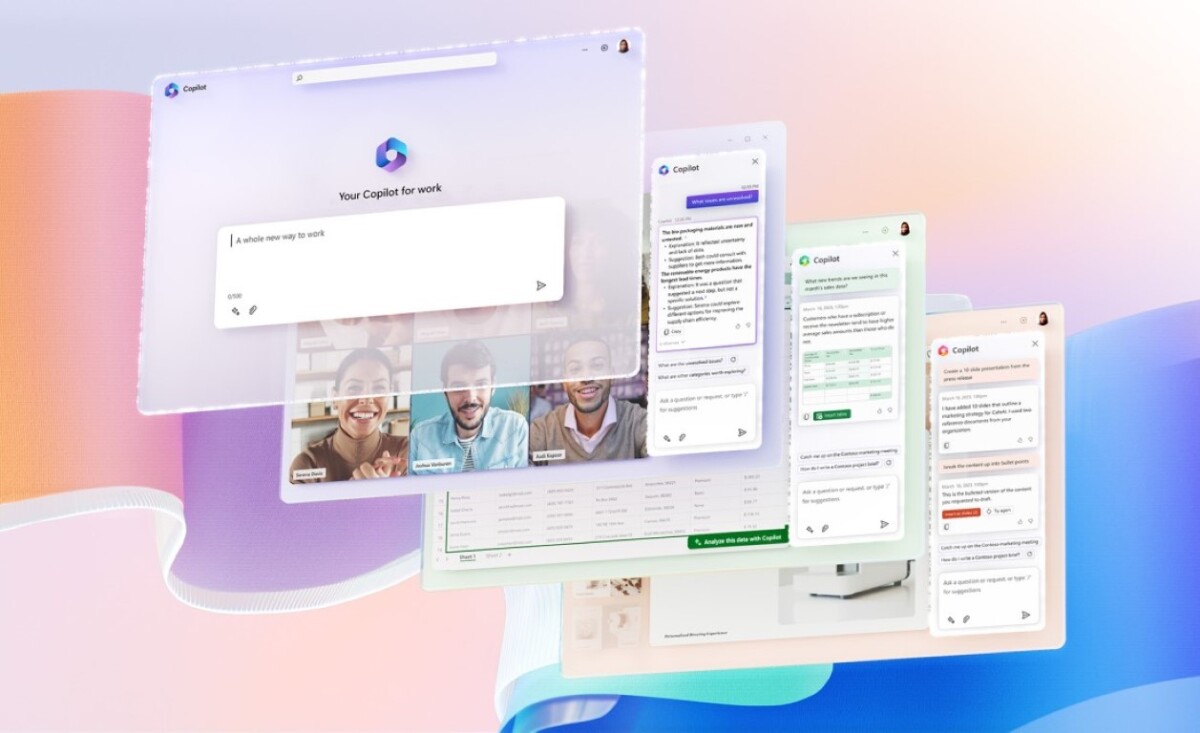
On Windows, the NPU can also be used to improve the performance of your games thanks to the AutoSR upscaling feature powered by AI. On Macs, the Neural Engine will be used to run Apple Intelligence, Apple’s AI assistant integrated into every facet of the system.
We’re still in the early days of local AI, so an internet connection is still needed to run some of these features. But NPUs will become faster and more accurate, opening the door to other applications and uses to improve productivity and creativity on laptops. That’s their promise, anyway.
Source: www.frandroid.com